Construction and Retrospective Validation of Structure-Based Virtual Screening Protocols to Identify Potent Ligands for Human Adrenergic Beta-2 Receptor.
Teks penuh
Gambar
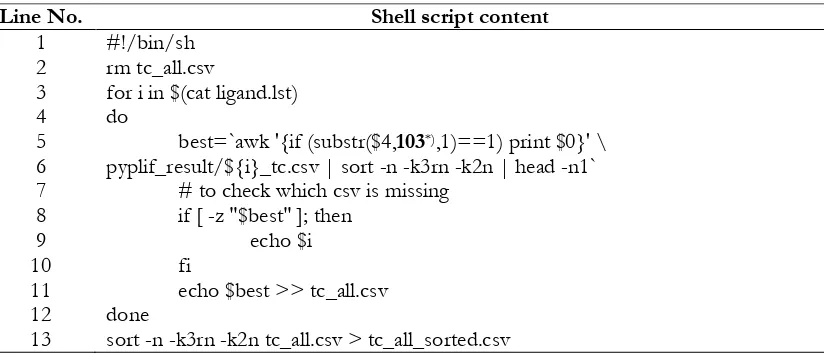

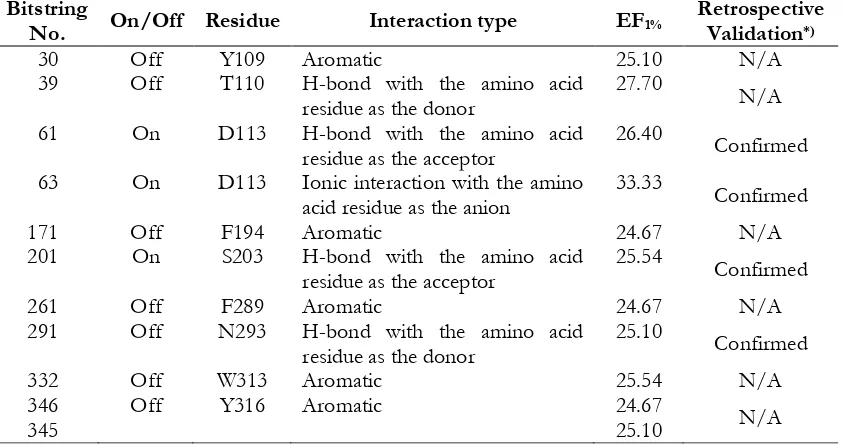
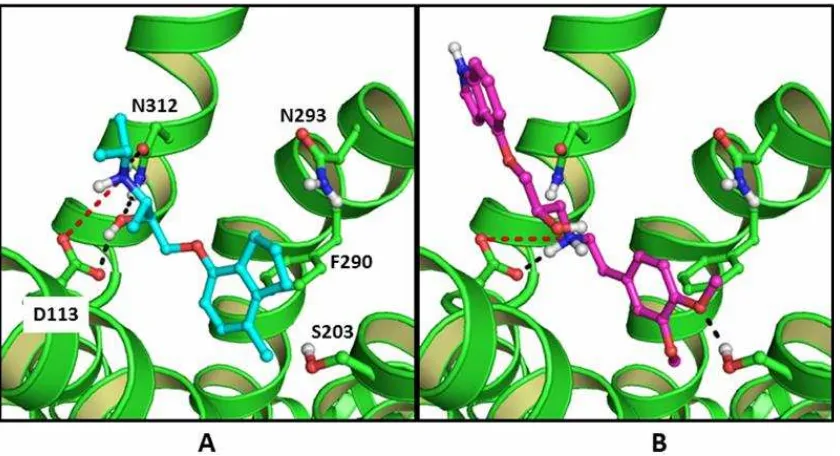
Dokumen terkait
Dari hasil penelitian diperoleh hasil bahwa: (1) Implementasi Corporate Governance BUMN listed lebih baik daripada non listed; (2) Rasio profitabilitas (marjin laba operasi)
Peserta lelang yang diundang agar membawa dokumen asli atau dokumen yang dilegalisir oleh pihak yang berwenang dan copy 1 ( satu ) rangkap sesuai dokumen pada isian kualifikasi
Penelitian yang berjudul “TRANSFORMASI KOMUNITAS PUNK di Condong Catur Yogyakarta dalam Prespektif Modal Sosial” ini memiliki tujuan penelitian,
Pasta gigi yang mengandung propolis ini terbukti efektif dalam terapi awal gingivitis ringan dengan rata- rata pengurangan skor indeks gingiva sebelum dan sesudah adalah 0,40.. ±
2 Keter ampil an 4.3.1 Membuat laporan hasil diskusi mengenai sistem tata nama makhluk hidup (C6) Penuga san Instru men Penilaian Tugas Terlampir Terlampir.. *) Ketentuan:..
Konstruksi-konstruksi mental tersebut adalah aksi (action), proses (process), objek (object), dan skema (scheme) yang disingkat dengan APOS. Sering sejumlah konstruksi
CHAPTER IV FINDING OF THE RESEARCH 4.1 The Content of Lesson Plan Written by the English Teachers of Senior High Schools in Kudus in the Academic Year 2012/2013 .... xiii
responsible for implementing malaria control interventions through Primary Health Care (PHC) approach under the Directorate of Communicable Disease Control. With the aim of achieving